Ingesting API Data in Google BigQuery the Serverless way!
Introduction
A few days ago I wrote 1st part of this blog. Where I talked about the concept that how can we design serverless data ingestion from API data either as streaming or batch pipeline. In this blog, we will see the code and configuration for the entire pipeline.
Let's get started.
Design
In the previous blog, we discuss pattern 1 where we are ingesting data from API and Inserting it into BigQuery In real-time. We will use this pattern for showing code and configuration.
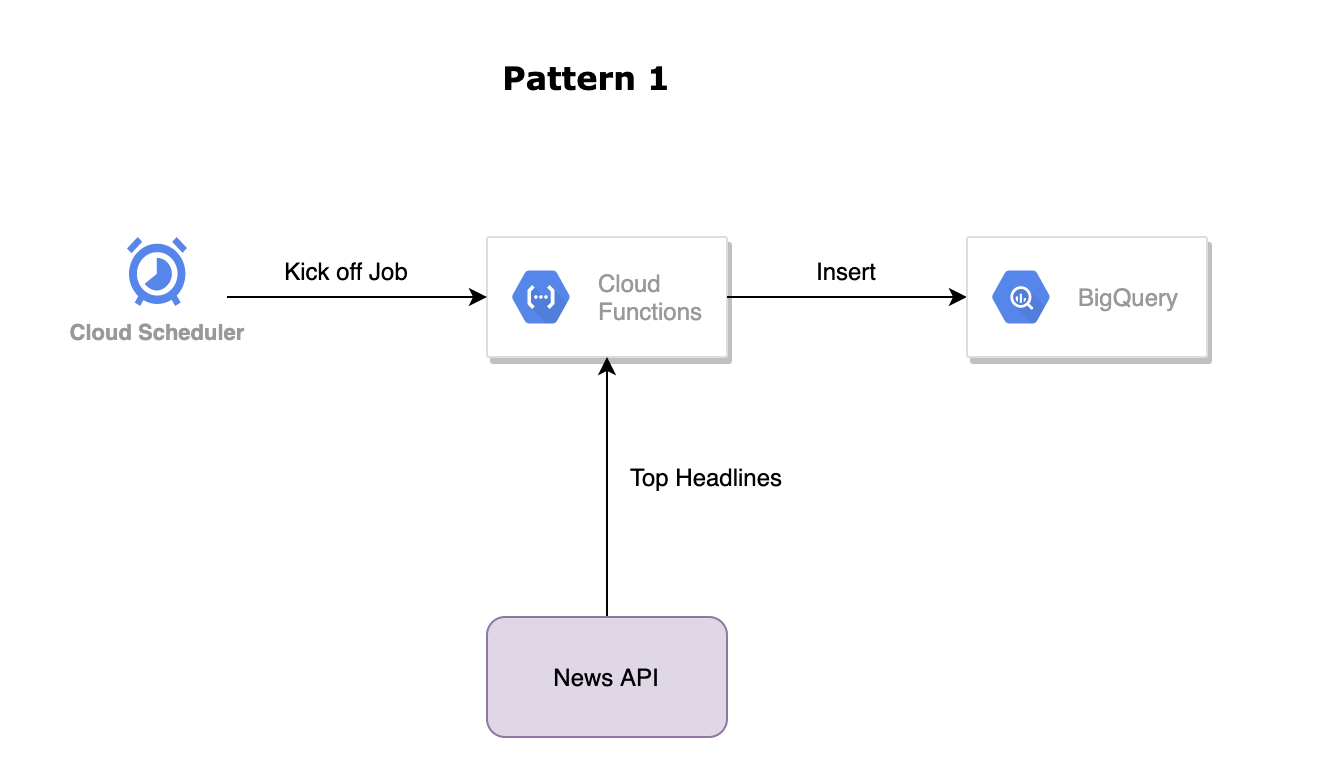
Cloud Workflow Yaml Configuration
- Cloud workflow is a serverless offering from GCP that allows us to design workflow that can execute our multistep pipeline as we design. If some steps get failed we can stop or take the necessary steps to alert system operators to know about failure.
- In our case, we are initializing some variables that we will use later in configurations such as project, datasetId, tableId, region, and SQL query.
- As per our design, we will execute a cloud function that will ingest data from News API to grab the top headlines.
- After ingesting data in cloud function we write that data as a streaming insert to our bigquery.
- Then we execute the SQL query job to compute some metrics and write the result into a result table called “daily_top_headlines_by_source”. we are making a call to the ComputeMetrics sub-workflow & we are also waiting until the job gets finished so that we can say it was successful or unsuccessful.
- After that, we log out our result of the final step.
Cloud Function API Ingestion Logic
- Let's deep dive into Our Cloud Functions logic. Cloud Functions are serverless functions that allow developers to write lightweight logic and execute in various language/execution environments such as Java, nodejs, python, etc.
- For our example, I am writing our lightweight logic in Java. I have used HTTP client make an HTTP request to news headline api and grab the response as JSON.
- Once JSON response is recieved i am using ObjectMapper to read and convert JSON response to JsonNode.
- JsonNode gives us the flexibility to extract the records and traverse each record and finally grab the important information such as source, author, title, description and so on.
- We will create a rowContent object that will store map data with key and value.
- Finally with BigQuery API we will insert row content to target big query table in streaming fashion.
HTTP Client Code
POM file
- In order to write HTTP client to grab news data and convert JSON to rowContent object and finally write to BigQuery table, we need to add some libraries in our POM.xml file.
- We have used 2 libraries, google cloud bigquery which provides rowContent Insert method into BigQuery, and Jackson-databind which provides Object mapper and JsonNode to read JSON response and traverse the JSON data.
- HTTP Client is used from Java 11 SDK and our execution environment support java 11 so no need to add anything in POM.xml.
BigQuery Destination Table
- Once the cloud function executes successfully we can see the result inserted result in the bigquery table.
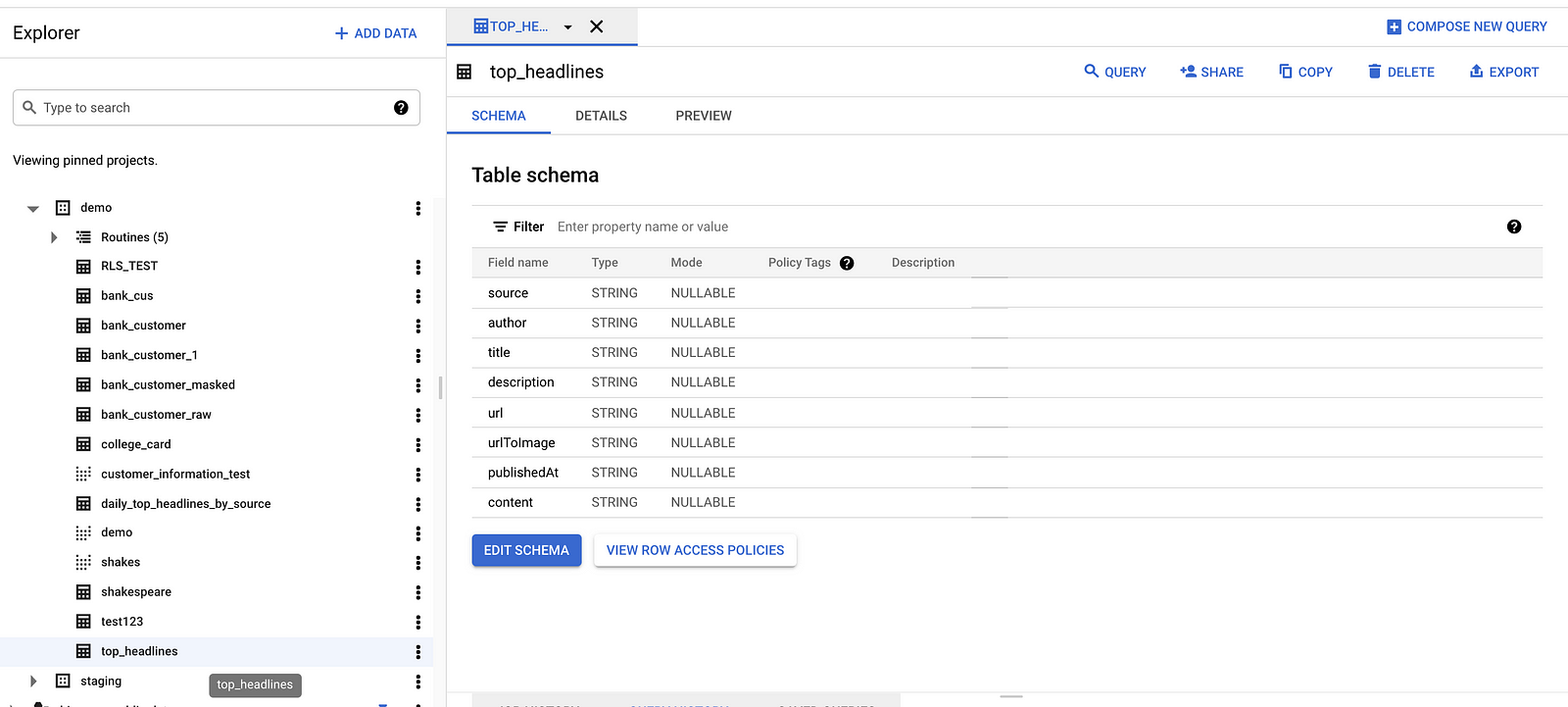
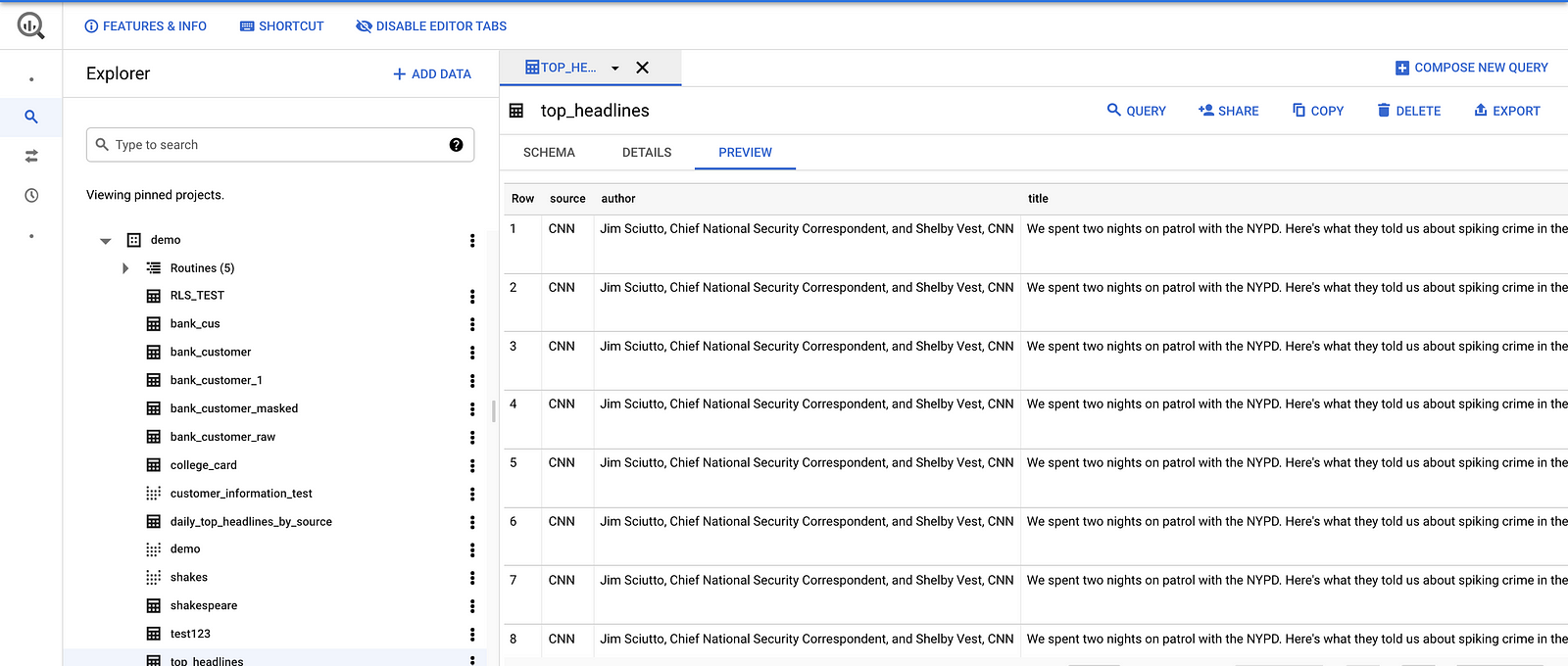
Computed Metrics
- Now as per design 2nd step is to compute the metrics and write it to another table called daily_top_headlines_by_source.
- We will run the below query and insert the result into our final table “daily_top_headlines_by_source” using bigquery query job that we have configured in cloud workflow.
source,count(*) as total_published
FROM
`avid-subject-309421.demo.top_headlines`
GROUP BY
source
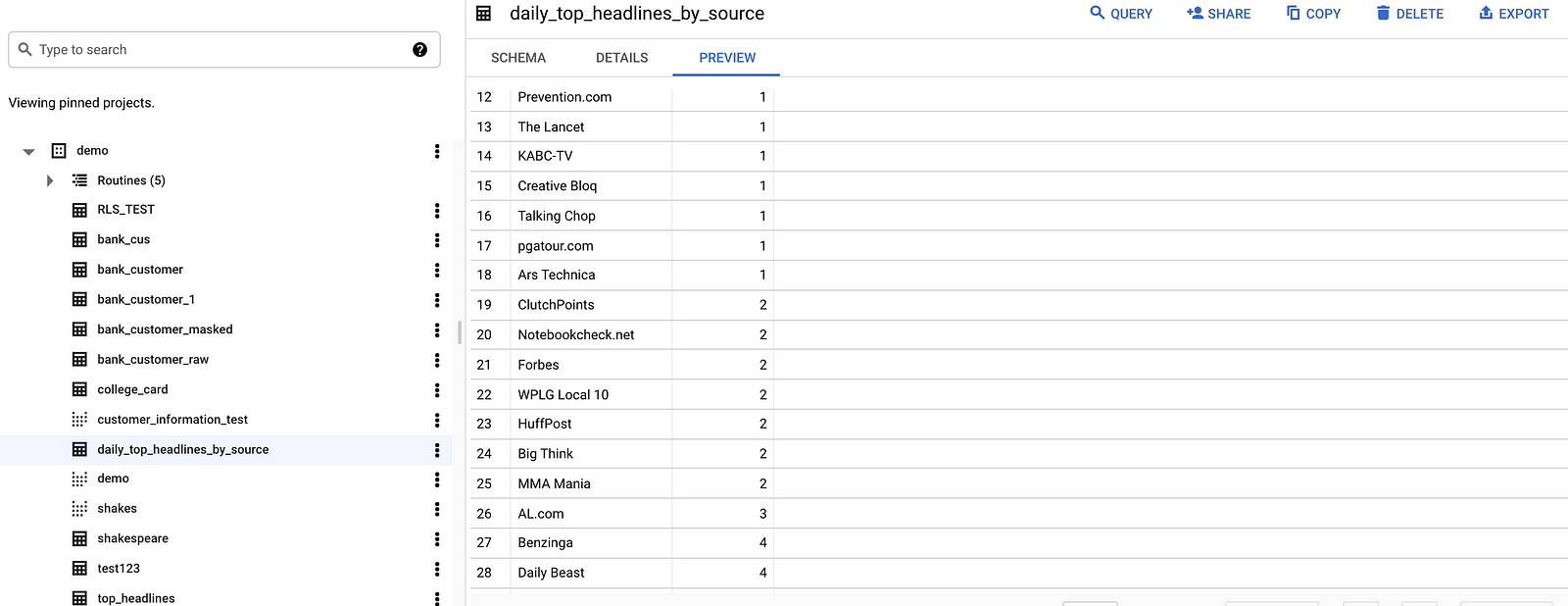
Conclusion
In Google Cloud, we can design and build no-ops pipelines very easily with serverless offerings. This blog just shows one of the use cases and barely touches the surfaces. But as your business getting started and you can't afford to have a big engineering team serverless and pay as you go is a savior for your business.
Don't treat Cloud as On-prem and take full advantage of the no-ops data pipeline.
Happy Pipeline Building! ✌️
Comments
Post a Comment